Machine learning and artificial intelligence technology are ubiquitous, but their use in medicine is lagging.
Every time we browse the internet, like a photo on social media, or look up directions on your phone, we are giving away personal data.
Online platforms such as YouTube, Facebook and Google use this personal data and feed it to machine learning algorithms to train automated programs to learn about our spending habits for targeted advertising or improving facial recognition software through the photos we post online.
We currently already use machine learning for common tasks such as junk email filtering, virtual assistants like Siri or Alexa, so why isn’t it used in healthcare more?
Machine learning has the potential to revolutionise healthcare by aiding in disease prediction and diagnosis, however uptake by the medical community has been slow. Research into the use of machine learning in asthma prediction is limited, albeit increasing, but there are no currently validated machine learning models to predict asthma in children.
Machine learning, as its name suggests, can be defined as giving “computers the ability to learn without being explicitly programmed”.1 While it might seem like a new technology, the concept of machine learning has been around as early as the 1950s with a paper by Alan Turing introducing “the Imitation Game”, a test to determine if machines could think.2 Turing used chess as an example of how machines could learn, and this was realized in 1996 when the supercomputer Deep Blue designed by IBM beat reigning chess world champion Garry Kasparov.
In the last couple of decades, the advent of technological advances has brought about increased computing speeds, processing power, and data storage capabilities leading to a surge in the use of machine learning in industries ranging from manufacturing, banking and bakeries. With the availability of vast amounts of health data collected through electronic medical records, opportunities for the development of machine learning algorithms in the field of medicine are ripe for the undertaking.
Machine learning is currently used in various fields of medicine, most prominently in areas where imaging is used for disease diagnosis and management such as radiology and oncology. For example, commercial systems for medical imaging in cardiology3 or to identify melanomas are readily available and in use.4
Additionally, there has been a surge in machine learning tools as a result of the covid pandemic.5 In fact, covid was initially also identified through an artificial intelligence platform prior to the World Health Organization reporting this. A recent search of the US FDA’s list of approved artificial intelligence and machine learning-enabled medical devices did not reveal any respiratory-related tools unless linked to radiology.
Most machine learning models follow a typical development path. The key first step is having a sufficiently large representative dataset to address the research or clinical question of interest. This dataset is then split into a training and test dataset and used to teach or train the model (see separate box). Training of the model is done using various algorithms, and depending on the question to be addressed, may be classified as supervised or unsupervised learning. In supervised learning, human input is required where the input data is labelled together with the outcome of interest with the purpose of identifying or predicting the outcome in a new dataset.
As might be expected from the name, unsupervised learning requires less human input and can use raw, unlabelled data to train a model with main tasks including classifying and identifying trends in the dataset. For the example of using machine learning to predict childhood asthma, in the supervised learning approach the model would be trained using a dataset where there is a representative sample of patients with asthma, and these patients are identified and labelled. Whereas using the unsupervised learning approach, the model would be asked to work out differences between groups of people within the dataset without identification of whether they were asthmatic or not, and the model may group individuals based on similar symptoms or risk factors.
The ability to predict childhood asthma has been a goal of respiratory doctors and researchers over the last 20 years. Asthma diagnosis is difficult in young children. Wheeze is a symptom of asthma that presents in the first few years of life. Wheeze, however, is not indicative of asthma since causes of wheeze also include bronchiolitis, a common acute viral infection. Clinicians often use wheeze symptoms and medical history to provide an asthmatic status for young children. This may lead to misdiagnosis and mismanagement of disease.
As a result, various asthma predictive indexes in childhood have been developed. While most of these have a high specificity or negative predictive value, the specificity or ability to accurately predict the presence of asthma is modest and therefore not widely adopted in clinical practice.6 Asthma is a disease with many risk factors, both environmental and genetic. These risk factors, which are termed “features” in machine learning language, may be incorporated as predictors during model development, with the advantage of machine learning being able to handle a large number of features and their interactions, unlike traditional statistical models.7
In a review published earlier this year, we discuss the machine learning models that have been undertaken in childhood asthma prediction and found only seven published papers.8 We also found several limitations in the published studies. These limitations, which are common across the field of machine learning, include a lack of external validation and not having a sufficiently large representative sample size. Validation of the machine learning model developed using the training dataset is essential for ensuring that the model can predict what it is supposed to. Most studies, however, only validate internally using the same dataset used to develop the model, but if it can be demonstrated that the model works just as well in a separate dataset, this indicates the model is generalisable to other similar groups.
Having a dataset that is appropriately representative of the outcome has also shown to be lacking in various fields of machine learning. For example, a limitation of the previously mentioned melanoma detection software was that most of the training images were derived from fair-skinned patients, and therefore its accuracy in other ethnicities was unknown.4
Racial bias is known to be a problem in the field of artificial intelligence (AI) with numerous examples in the technology field of algorithms being racist. By understanding how machine learning models work and the requirements and associated limitations, we can, however – like the machines – learn and build better.
A lack of understanding as to how machine learning algorithms work may be a contributing factor to why uptake of machine learning within not only respiratory medicine, but healthcare in general, is low.
A recent report highlighted this by showing the number of job advertisements requiring AI skills in the US, with healthcare being the second lowest industry, just above construction. Clinicians may be reticent to adopt machine learning in their practice due to mistrust and fear of liability should the wrong AI decision be made. Other major barriers are related to data access. As previously discussed, the development of reliable machine learning models is dependent on the availability of high-quality training data. Concerns regarding the quality of electronic health records and the ability to pool them across various health systems, coupled with strict privacy regulations for access to health care data have hindered machine learning advancement in healthcare. Similarly, in Australia, regulatory and legal bodies have not kept up with the rapid development of machine learning ( AI in health care: Australia in danger of lagging behind | InSight+ (mja.com.au)).
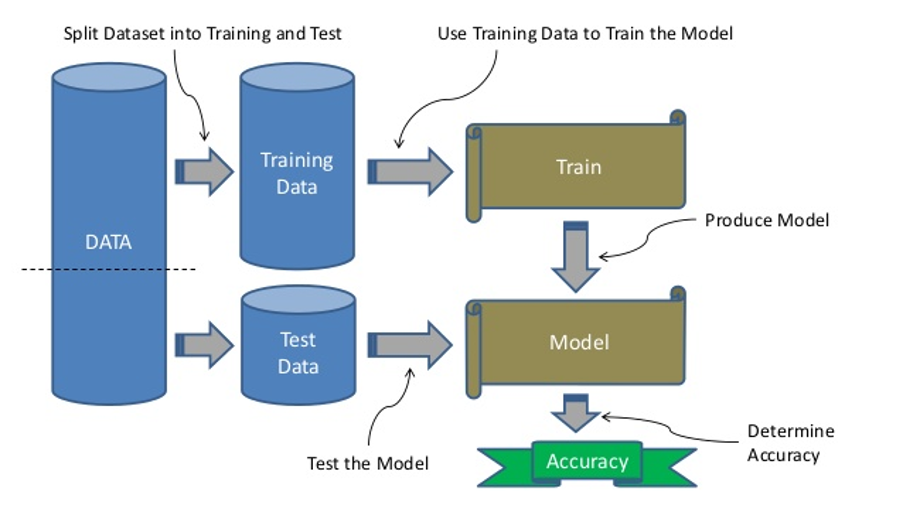
Development of a machine learning model
Main steps include having a dataset that is split into training data to teach the model, and test data to validate the model that has been developed using the most suitable machine learning algorithm. The accuracy of the model is then determined using various predictive performance measures, such as reporting of the sensitivity, specificity or area under the curve.
Conclusion
Using data from a general Australian population, we aim to use machine learning to develop a personalised prediction score for childhood asthma using family history, environmental and genetic risk factors as predictors. If this is successful and can predict asthma with high accuracy, next steps would be to incorporate the model into an online app or software for doctors to use during a clinic visit to generate a personalised prediction asthma risk score for the individual patient.
However, to get to this stage would require extensive consultation with health providers, community end-users and would software and application developers working together with researchers, clinicians and asthma patients to implement this tool within a clinical setting.
For machine learning to be beneficial to patients and society, it requires the input of clinicians who understand the health issues working together with data scientists. If doctors are aware of the limitations of these model, they are able to make informed decisions as to whether a specific device or software is suitable for their patient population. Only if clinicians are part of the conversation can machine learning achieve its full potential in healthcare and implemented into clinical practice to predict risk of diseases such as asthma.
Dr Rachel Foong is the recipient of the 2021 Asthma and Airways Career Development Fellowship, jointly funded by the National Asthma Council Australia and the Thoracic Society of Australia and New Zealand.
References
1 Mitchell T. Machine Learning. McGraw Hill, New York, 1997.
2 Turing AM. I.—Computing Machinery And Intelligence. Mind. 1950; LIX: 433-60.
3 Raimondi F, Martins D, Coenen R, Panaioli E, Khraiche D, Boddaert N, Bonnet D, Atkins M, El-Said H, Alshawabkeh L, Hsiao A. Prevalence of Venovenous Shunting and High-Output State Quantified with 4D Flow MRI in Patients with Fontan Circulation. Radiology: Cardiothoracic Imaging. 2021; 3: e210161.
4 Winkler JK, Sies K, Fink C, Toberer F, Enk A, Deinlein T, Hofmann-Wellenhof R, Thomas L, Lallas A, Blum A, Stolz W, Abassi MS, Fuchs T, Rosenberger A, Haenssle HA. Melanoma recognition by a deep learning convolutional neural network—Performance in different melanoma subtypes and localisations. European Journal of Cancer. 2020; 127: 21-9.
5 Chen J, See KC. Artificial Intelligence for COVID-19: Rapid Review. J Med Internet Res. 2020; 22: e21476-e.
6 Pinart M, Smit HA, Keil T, Bousquet J, Antó JM, Lødrup-Carlsen KC. Systematic review of childhood asthma prediction models. European Respiratory Journal. 2015; 46: PA4513.
7 Rajkomar A, Dean J, Kohane I. Machine Learning in Medicine. New England Journal of Medicine. 2019; 380: 1347-58.
8 Patel D, Hall GL, Broadhurst D, Smith A, Schultz A, Foong RE. Does machine learning have a role in the prediction of asthma in children? Paediatric Respiratory Reviews. 2022; 41: 51-60.